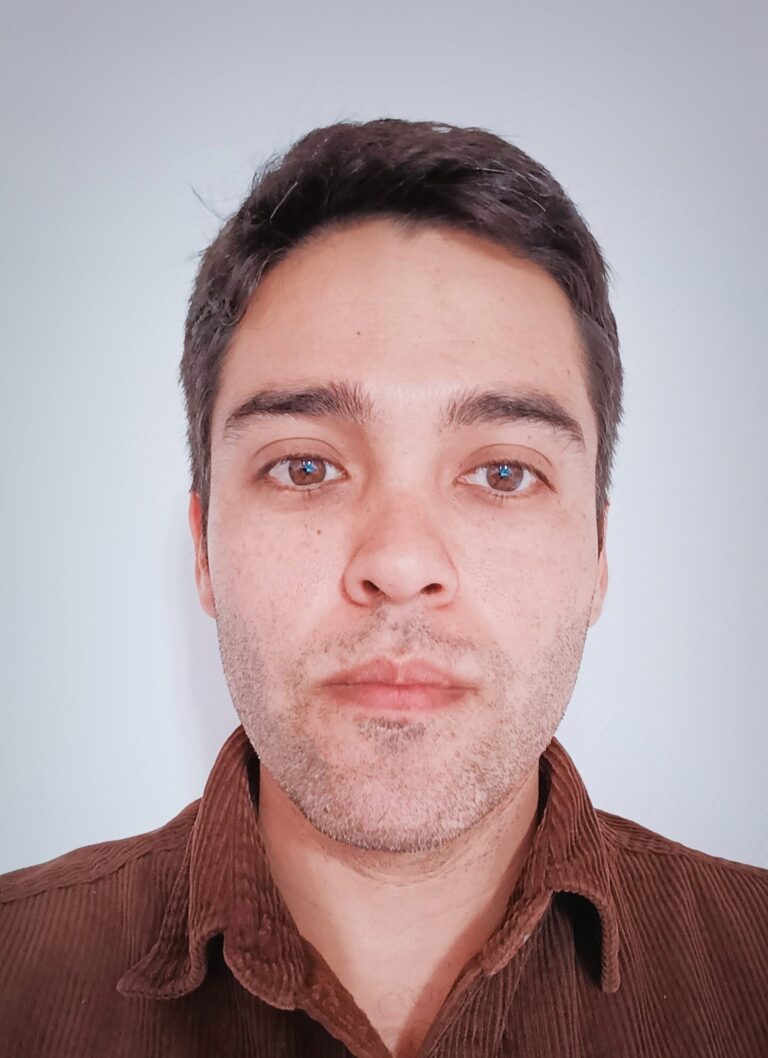
About my academic career
B.Sc in Biochemistry @ University of Antofagasta, Chile
Work experience: Research in Optimization of chemical processes and analytical chemistry.
2013-2015:
Center of Scientific studies of Chile, Valdivia, Chile
2016-2019:
Albemarle Corporation, Antofagasta, Chile
Erasmus Mundus Joint Master, Excellence in Analytical Chemistry (EACH) @ University of Tartu, Tartu, Estonia and University Claude Bernard Lyon1, Lyon, France. Scholarship Holder.
Master thesis: Development of a methodology for in-line and real-time monitoring CO2 and NH3 concentrations in strong ammonia waters using Raman spectroscopy.
About my thesis - Reaction process screening and monitoring
In chemical process development, optimizing reactions is crucial, aiming to improve factors like product yield and quality. Traditional methods involve many experiments with different conditions, making it time-consuming and expensive. The use of inline analytical techniques, including NIR, FTIR, Raman, HPLC, MS, and even NMR, and design of experiments (DoE) has represented a significant step forward, enabling the characterization of the reaction products in real-time and decreasing the required number of experiments. Still, reaction screening is very time-consuming, as many variables need to be investigated, including reaction temperature, pressure, concentrations, reaction time, etc. In electrosynthesis, the process is even more complicated due to complex variables like electrolyte type, electrolyte concentration, and working potential.
We will work on automated systems for electrosynthesis screening and optimization. These systems will use math-based algorithms to guide continuous electrosynthesis processes automatically toward set goals, such as maximizing product yield, productivity of a transformation, or a desired product distribution. To monitor the reactions, inline and real-time spectroscopic instrumentation will be used, and the data collected will provide instant feedback to the system. This way, the system can control key parameters like the reactor settings, reagent inputs (such as flow rates and concentrations), and process conditions (pressure, temperature, etc.) directly. Instead of DoE, evolutionary algorithms (such as deep reinforcement learning or Bayesian models) will be tested.
Optimization based on mathematical optimization algorithms reduces human intervention, resulting in a significant reduction of workload and acceleration of development processes. Furthermore, it helps with sustainability due to the reduced number of experiments required during the optimization process.